BigSurv23 (Big Data Meets Survey Science)
BigSurv23 (Big Data Meets Survey Science)
En la Conferencia Internacional BigSurv23 (Big Data Meets Survey Science) debatirán destacados científicos y profesionales del mundo sobre los temas más innovadores en la ciencia de los datos y las encuestas, así como las ciencias computacionales aplicadas a las ciencias sociales.
La conferencia magistral virtual estará a cargo del Dr. Juan M. Lavista Ferres, VP, Chief Data Scientist del Laboratorio Microsoft AI for Good.
BigSurv23 es una iniciativa global y multidisciplinaria, que se realizará por primera vez en Latinoamérica, teniendo como sede a la Universidad San Francisco de Quito -USFQ-.
En el marco de BigSurv23 se realizarán dos eventos vibrantes y complementarios:
El primer evento complementario es el Data Challenge sobre el tema de la desnutrición crónica infantil. Su objetivo es usar la ciencia de los datos para el bien común. Los participantes recibirán becas y premios.
El segundo evento complementario son los cursos cortos de actualización.
Los cupos en BigSurv23 y sus eventos complementarios son limitados.
Para mayor información: bigsurv@aenu.ec
REGISTRO Y PAGO
Puedes realizar tu registro en el siguiente link:
Registro
Thursday 26th October
**Agenda sujeta a cambios, para mayor información consulta la página oficial: https://www.bigsurv.org/program23
Hour |
Activity |
---|---|
08:30 - 18:30 |
Download the BigSurv23 Program in PDF format here. |
09:00 - 12:00 |
Short Course 1: Data Integration, instructor: Trivellore Raghunathan ("Raghu") |
12:00 - 13:00 |
Lunch (on your own) |
13:00 - 16:00 |
Short Course 3: Unlocking the Superpowers of Advanced Machine Learning Models for Social Scientists: From Lassos to Boosts to Nets! |
17:00 - 18:45 |
Welcome and Opening Plenary Panel: My Chatbot is Hallucinating about your Digital Trace. A discussion about the future of computational social sciences in the era of AI. |
18:45 - 20:30 |
Welcome Reception (Room: Shakespeare Theatre Hall, USFQ |
Friday 27th October
**Agenda sujeta a cambios, para mayor información consulta la página oficial: https://www.bigsurv.org/program23
Hour |
Activity |
---|---|
08:00 - 17:00 |
Download the BigSurv23 Program in PDF format here. |
08:30 - 17:30 |
Poster Session (actively presented 8:30-10:00)Chair: Ana Lucía Córdova Cazar (Universidad San Francisco de Quito) ML Applications to Survey Quality Control and Fraud Detection Abstract 2023 Volatility and irregularity Capturing in stock price indices using time series Generative adversarial networks. Statistical learning methods to estimate sales forecasts for products that affect the supply chain of a mass consumption company in the city of Guayaquil Unraveling the Correlation between Perceived Issue Importance and Issue Salience On the Internet among Users with Different Media Repertoires Reliable Inference from Imperfect Data Optimism and cryptoasset ownership Technological Developments Influence the Cybercrime in Juja Sub-County |
10:00 - 11:30 |
Opening Keynote by Dr. Juan M. Lavista Ferres, VP, Chief Data Scientist of the Microsoft AI for Good Lab (Live presentation with Q&A) |
11:30 - 11:45 |
Coffee Break |
11:45 - 13:15 |
CONCURRENT SESSIONS A
|
11:45 - 13:15 |
CONCURRENT SESSIONS A
|
11:45 - 13:15 |
CONCURRENT SESSIONS A
|
11:45 - 13:15 |
CONCURRENT SESSIONS A
|
13:15 - 14:30 |
Group Lunch (University Restaurant) - Lunch tickets available |
14:30 - 16:00 |
CONCURRENT SESSIONS B
|
14:30 - 16:00 |
CONCURRENT SESSIONS B
|
14:30 - 16:00 |
CONCURRENT SESSIONS B
|
14:30 - 16:00 |
CONCURRENT SESSIONS B
|
16:00 - 16:30 |
Coffee Break |
16:30 - 18:00 |
CONCURRENT SESSIONS C
|
16:30 - 18:00 |
CONCURRENT SESSIONS C
|
16:30 - 18:00 |
CONCURRENT SESSIONS C
|
16:30 - 18:00 |
CONCURRENT SESSIONS C
|
19:30 - 21:30 |
Conference Dinner at Colonial Quito and Visit to the awe-inspiring Church of the Society of Jesus in Quito (Tickets Available here ) |
Saturday 28th October
**Agenda sujeta a cambios, para mayor información consulta la página oficial: https://www.bigsurv.org/program23
Hour |
Activity |
---|---|
08:00 - 17:00 |
Download the BigSurv23 Program in PDF format here. |
09:00 - 10:30 |
CONCURRENT SESSIONS D
|
09:00 - 10:30 |
CONCURRENT SESSIONS D
|
09:00 - 10:30 |
CONCURRENT SESSIONS D
|
09:00 - 10:30 |
CONCURRENT SESSIONS D
|
10:30 - 11:00 |
Coffee Break |
11:00 - 12:30 |
CONCURRENT SESSIONS E
|
11:00 - 12:30 |
CONCURRENT SESSIONS E
|
11:00 - 12:30 |
CONCURRENT SESSIONS E
|
11:00 - 12:30 |
CONCURRENT SESSIONS E
|
12:30 - 14:00 |
Group Lunch (University Restaurant) - Lunch tickets available |
14:00 - 15:30 |
CONCURRENT (ORGANIZED) SESSIONS F
Given the large volume of opinions people express on social media, a new lens exists for measuring public opinion as a supplement to traditional survey-based methods. But systematic differences between surveys and social media–in terms of how they are collected, processed, and analyzed–mean that there is no one-to-one translation between observations from each method. To make the best use of both types of data in concert, scholars need to better understand how they differ and how to translate between them. This panel compared data on attitudes toward Covid-19 vaccination, economic threat, and schooling from (1) probability-based surveys, (2) linked Twitter posts from a subset of survey respondents who consented to data linkage, and (3) a random sample directly from Twitter. Collectively, these papers will help identify which theoretical gaps between data streams are relatively easy to bridge and which require more scholarly attention. |
14:00 - 15:30 |
CONCURRENT (ORGANIZED) SESSIONS F
This session features five talks on CDC’s leveraging of data science and external data sources to adjust for total survey error in health surveys: |
14:00 - 15:30 |
CONCURRENT (ORGANIZED) SESSIONS F
Missingness is ubiquitous in surveys. Whether by design or accidental, missing data impedes statistical analyses and hinders generalizability of inferences. Imputation directly models the observed data, and weighting models the probability of a unit being observed: both somehow “learn” from observed data and usually assume that data is missing at random (MAR). When data is missing not at random (MNAR), the missing data mechanism needs to be modeled. This can be complex, rely on unverifiable assumptions and require deep insight into the missing data mechanism, or “How” the data is missing. Strategies for handling MNAR data leverage missing data patterns, or “Where” data is missing, reasons for missingness, or “Why” the data is missing, and external information. Although none are free of assumptions, some approaches can be more realistic and/or flexible than others. The proposed session includes four talks and a discussion from a demographically diverse group of scholars. |
14:00 - 15:30 |
CONCURRENT (ORGANIZED) SESSIONS F
The U.S. Census Bureau has long maintained frame-like data on individuals, households, businesses, and governments to support census and survey operations. However, these data are rarely used for enterprise-wide operations, despite abundant evidence of the value of integrating data to produce new and/or improved statistical products. The agency has established the Frames Program to meet the need for a modernized data infrastructure with a linked universe of information from which sampling can occur and statistical summaries directly produced. During this session, Census Bureau staff will summarize objectives and achievements of the nascent Frames Program, highlight the evolution of the existing Business, Job, and Geospatial Frames, detail efforts to establish a linkage infrastructure to better leverage these resources, and introduce the new enterprise frame: the Demographic Frame. Three presentations will detail initial assessments of the fitness for use of the Demographic Frame in census and survey taking. |
15:30 - 16:00 |
Coffee Break |
16:00 - 17:00 |
Special Networking Events |
17:00 - 17:45 |
Closing Remarks |
Sunday 29th October
**Agenda sujeta a cambios, para mayor información consulta la página oficial: https://www.bigsurv.org/program23
Hour |
Activity |
---|---|
08:30 - 14:30 |
Download the BigSurv23 Program in PDF format here. |
Data Challenge
BigSurv23 Data Challenge: Tackling Chronic Child Malnutrition
We are excited to introduce the BigSurv23 Data Challenge sponsored by REDNI, focused on addressing chronic child malnutrition through innovative data-driven solutions. Just as how previous BigSurv Data Challenges brought together teams to work on open data challenges, we aim to harness the power of data scientists, computer scientists, social scientists, and survey and big data experts from Ecuador and around the world to make a significant impact in the fight against child malnutrition in Ecuador.
Background Information:
The Challenge: Our goal is to combat chronic child malnutrition by leveraging data, insights, and digital tools. We want to understand the underlying factors contributing to this issue, identify at-risk populations, and develop strategies to improve the nutrition of affected children.
Data Sources: Participants in this data challenge will have access to a rich dataset provided by the sponsor institution, containing demographic information, nutritional data, and related information. You will also have access to survey data and other relevant sources.
The Teams: We will have multiple teams, each consisting of interdisciplinary participants who will collaborate to address specific aspects of the chronic child malnutrition challenge. Your team's composition will encourage diversity of thought and expertise.
Support from Experts: Throughout the data challenge, expert mentors in the fields of data science and nutrition will be available to guide and support the teams. Child nutrition experts, members of BigSurv23 scientific committee, USFQ professors, and data professionals will also be on hand to provide data expertise.
Presentation and Recognition: At the conclusion of the data challenge, each team will present their findings and proposed solutions to a panel of expert judges. Not only will this provide valuable exposure for your work, but the winning team will also have the opportunity to give a flash-talk during the BigSurv23 closing remarks.
Mentorsand Judges: We have assembled a distinguished group of mentors and judges, including nutrition experts from, members of the BigSurv Scientific Committee, experienced data scientists, and industry professionals.
Prizes:
1. A formal reward for the winner (1000 USD) and runner up (500 USD) will be awarded by the Data Challenge sponsor.
2. The School of Business of the USFQ will provide an academic recognition to the winners.
Application Process: If you're interested in participating in the BigSurv23 Data challenge, please apply directly via this link: https://aenu.ec/data-challenge/
Participation in the Data Challenge is free of charge.
Important Dates:
- Application Deadline: October 13, 2023
- Notification of Acceptance: October 18, 2023
- Data challenge Dates: October 25, 11 am - October 26, 10 am
Location: The data challenge will take place at the Main Hall of Universidad San Francisco de Quito (USFQ).
Refreshments: Lunch and coffee breaks will be provided.
Requirements: To participate, bring your enthusiasm, a personal laptop with relevant software (e.g., Python, R/R-Studio, Tableau), and a willingness to collaborate and innovate. WiFi will be available, and refreshments will be provided to keep your creative energy flowing.
Join us in this exciting data-driven challenge and contribute to the fight against chronic child malnutrition with the support of REDNI and the global data science community. Together, we can make a meaningful difference in children’s lives.
What we are looking for:
Blueprint of the Architecture and Data Flow: Participants are expected to provide detailed blueprints outlining the architecture and data flow for their proposed solutions. Describe how data will be collected, processed, and analyzed to tackle chronic child malnutrition effectively.
Methodology Sketches: Present sketches and outlines of the methodologies you plan to employ in the design and processing of data. Explain the techniques, algorithms, and statistical methods you intend to use to identify and combat malnutrition among children.
Realistic Demo: Participants should demonstrate a realistic implementation of their solution. This could include a prototype or proof of concept that showcases how your approach can make a tangible impact on addressing chronic child malnutrition. Real-world applicability and feasibility are key.
Implementation Outlook: Provide insights into how your solution could be practically implemented. Consider factors like scalability, sustainability, and integration with existing nutrition programs and initiatives supported by the sponsor institution.
Follow-up: Ideas on how to advance the DataNutriNet (Red de Jóvenes Analistas de Datos en contra de la Desnutrición Infantil)
Participants in this challenge will have the opportunity to collaborate with experts and access valuable resources to enhance their solutions.
By participating in this data challenge, you will contribute to a noble cause and potentially make a significant impact on the well-being of children affected by chronic malnutrition. We encourage creative and data-driven approaches that can lead to actionable insights and effective interventions in this critical area.
You can find the contest rules here: https://www.bigsurv.org/Rules
Thanks to the support of:
Cursos cortos
**Agenda sujeta a cambios, para mayor información consulta la página oficial: https://www.bigsurv.org/shortcourses
To register and pay for short courses, please go to:
https://aenu.ec/registro/
Short Course 1: Data Integration
Instructor: Dr. Trivellore Raghunathan (“Raghu”)
Description:
The data landscape has changed tremendously. Until a few years ago, sample surveys were the primary sources of information but with the ability to harness data from many other sources have become available. These include spatial observations, administrative sources, sensor data, business transactions and social media, to just name a few. These “found data” provide unique opportunities to blend information from multiple sources to harness inferences about the population of interest to address societal problems. This short course will cover important challenges such as harmonization and comparability of measurements across various sources, methods to combine information, modeling challenges and framework needed to evaluate the validity and reliability of estimates derived from such combined sources. Several case studies will be used to illustrate the challenges, opportunities and benefits.
Short Course 2: Fundamentals of Data Science
Instructor: Dr. Juan Esteban Díaz Leiva
Description:
Data are everywhere and come in overwhelming quantities. Thus, being able to extract relevant information from them has become an essential ability. Machine learning allows us to do this by granting us “superpowers”, such as seeing in more than 3 dimensions or recognizing patterns when dealing with millions of variables. Here we will introduce this branch of artificial intelligence, briefly review its main areas, and finally focus on regression and clustering, which are two of the most used tools from supervised and unsupervised learning, respectively.
Short Course 3: Unlocking the Superpowers of Advanced Machine Learning Models for Social Scientists: From Lassos to Boosts to Nets!
Instructors: Dr. Trent D Buskirk & Dr. Adam Eck
Description:
Social scientists and survey researchers are confronted with an increasing number of new data sources such as apps and sensors that often result in complex data structures that are difficult to handle with traditional modeling methods. At the same time, advances in the field of machine learning (ML) have created an array of flexible methods and tools that can be used to tackle a variety of modeling problems. Against this background, this course discusses advanced ML frameworks, methods and models such as regularization methods, ensemble approaches to learning and deep learning models. The course aims to illustrate these concepts, methods and approaches from a social science perspective in an accessible way so that researchers can apply these methods in their own work to unlock insights. Code examples will be provided using both R and Python and will be available to attendees. The course assumes basic familiarity with fundamental machine learning methods like regression, logistic regression and tree-based models.
Training Session: Hands-on training to select a two-stage gridded population sample using free, user-friendly tools
Instructors: Dr. Dana R Thomson & Dr. Dale Rhoda
Description:
Household surveys in countries with an outdated census, or in complex urban settings with mobile or informal populations can be implemented with an improved sample frame based on modelled gridded population estimates. This hands-on training will briefly introduce survey practitioners to the emerging field of gridded population sampling before guiding attendees through two hands-on activities. The activities are based on free, easy-to-use tools – GridSample and GeoSampler – so no special programming or GIS skills are required to attend this session. In the first activity, attendees will generate a sample frame from gridded population data and select primary sampling units with probability proportional to size (GridSample). In the second activity, attendees will randomly sample structures (GeoSampler). Further instruction will be provided about questions to include in the survey questionnaire that allow adjustments for households-per-structure in the sample weights, and production of digital/paper maps that enable easy navigation for field workers. The training is based on the recently published manual on “Designing and Implementing Gridded Population Surveys.”
INSTRUCTORS
Trivellore Raghunathan (“Raghu”)
Professor of Biostatistics at the School of Public Health, Research Professor of Survey Methodology at the Institute for Social Research, University of Michigan. He is also Research Professor at the Joint Program in Survey Methodology, University of Maryland. His research interests are in the analysis of incomplete data, multiple imputation, Bayesian methods, design and analysis of sample surveys, combining information from multiple sources, small area estimation, confidentiality and disclosure limitation, longitudinal data analysis and statistical methods for epidemiology. He has developed a SAS based software for imputing the missing values for a complex data set and can be downloaded from www.iveware.org. He is a Fellow of American Statistical Association, received Richard Remington Award from American Heart Association and Monroe Sirken Award for his contributions to Survey Methodology.
Juan Esteban Díaz Leiva
Director of the USFQ Data Science Institute, director of the Master Program in Data and Business Management and Professor of Operations Management at Universidad San Francisco de Quito. He was awarded a PhD in Business and Management by the University of Manchester. He also holds a master's degree in Food and Resource Economics from Bonn University and a Food Engineering degree from Universidad San Francisco de Quito. He is an expert in evolutionary computation, automatic algorithm design and configuration, multiobjective optimisation under uncertainty and artificial intelligence. He also has multiple publications in high-impact journals and a is a consultant in areas such as artificial intelligence, business analytics, data science, among others.
Trent D. Buskirk
Trent D. Buskirk, Ph.D. is the Novak Family Distinguished Professor of Data Science and outgoing Chair of the Applied Statistics and Operations Research Department at Bowling Green State University. Dr. Buskirk is a Fellow of the American Statistical Association and his research interests include big data quality, recruitment methods through social media, the use of big data and machine learning methods for health, social and survey science design and analysis, mobile and smartphone survey designs and in methods for calibrating and weighting nonprobability samples and fairness in AI models and interpretable ML methods. Recently, Trent served as the President of the Midwest Association for Public Opinion Research in 2016, the Conference Chair for AAPOR in 2018 and is currently part of the scientific committee for the BigSurv23 conference. Trent also serves as an Associate Editor for Methods for the Journal of Survey Statistics and Methodology. When Trent is not geeking out over data science, big data or survey methodology, you can find him playing a competitive game of Pickleball!
Adam Eck
Adam Eck is an Associate Professor of Computer Science and Chair of the Data Science Integrative Concentration at Oberlin College where he leads the Social Intelligence Lab. Adam's research interests include interdisciplinary applications of artificial intelligence and machine learning to solve real-world problems, such as data science and machine learning for improving data collection and analysis in the computational social sciences (e.g., Survey Informatics) and public health, as well as decision making for intelligent agents and multiagent systems in complex, uncertain environments.
Dana Thomson
Dana Thomson is a pioneer in the field of gridded population household surveys. She also coordinates the IDEAMAPS Network, a global initiative that integrates "slum" mapping traditions to map deprived urban areas routinely and accurately at scale. Her other work includes improving the accuracy of gridded population datasets, measuring "slum" upgrading in ways that incentivize community participation, and co-developing data trainings for "slum"-based researchers and advocates. Dr. Thomson is a consultant and visiting researcher at the University of Twente (Netherlands).
Dale Rhoda
Dale Rhoda is a statistical consultant and expert on design & analysis of household surveys for public health. In recent years, he led the statistical aspects of updating the World Health Organization guidelines on vaccination coverage surveys. He regularly coordinates design and analysis of large country-wide surveys in Africa and Asia. Dr. Rhoda is currently interested in data entry errors with touchscreen devices, how entry errors propagate through analysis workflows, using gridded population datasets as survey sampling frames, characterizing missed opportunities for vaccination, and designing survey samples with both design- and model-based estimation in mind.
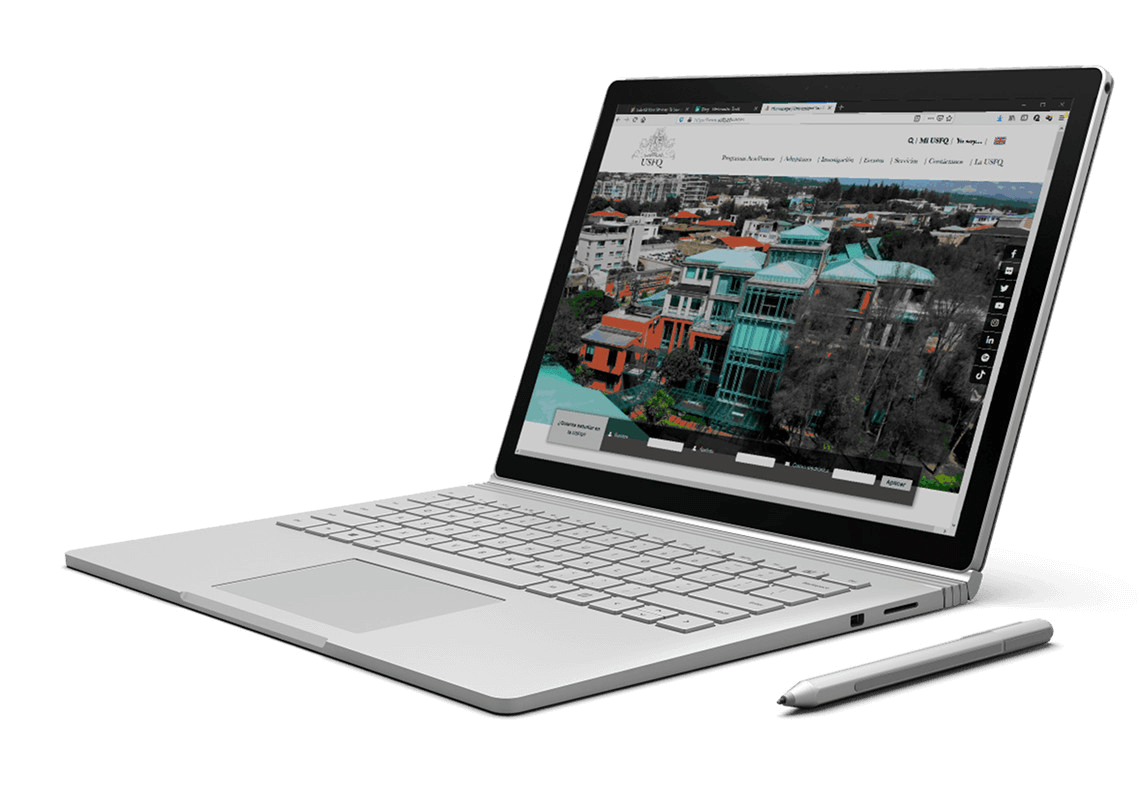